Autonomous Vehicles
Overview
Autonomous perception requires high-quality environment sensing in the form of 3D bounding boxes of dynamic objects. The primary sensors used in automotive systems are light-based cameras and LiDARs. However, they are known to fail in adverse weather conditions. Radars can potentially solve this problem as they are barely affected by adverse weather conditions. However, specular reflections of wireless signals cause poor performance of radar point clouds.We introduce Pointillism, a system that combines data from multiple spatially separated radars with an optimal separation to mitigate these problems. We introduce a novel concept of Cross Potential Point Clouds, which uses the spatial diversity induced by multiple radars and solves the problem of noise and sparsity in radar point clouds. Furthermore, we present the design of RP-net, a novel deep learning architecture, designed explicitly for radar’s sparse data distribution, to enable accurate 3D bounding box estimation. The spatial techniques designed and proposed in this paper are fundamental to radars point cloud distribution and would benefit other radar sensing applications.
Publications
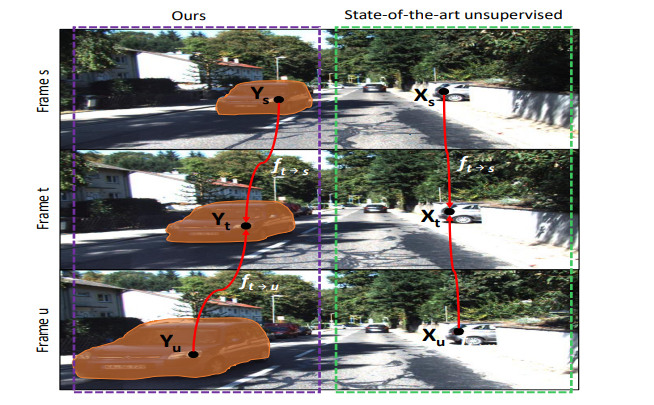
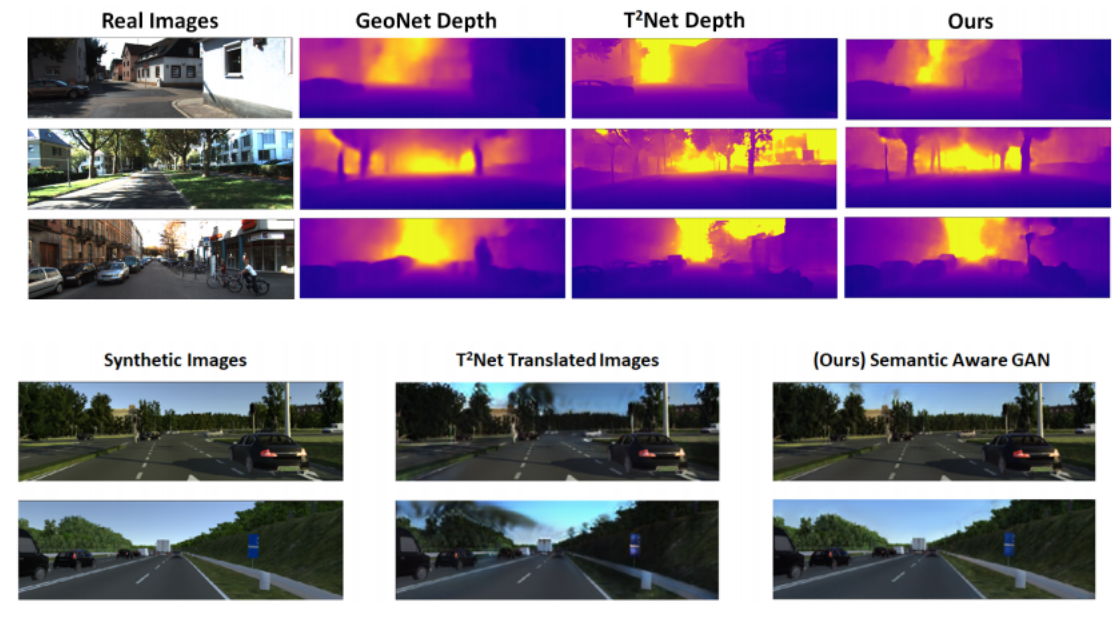
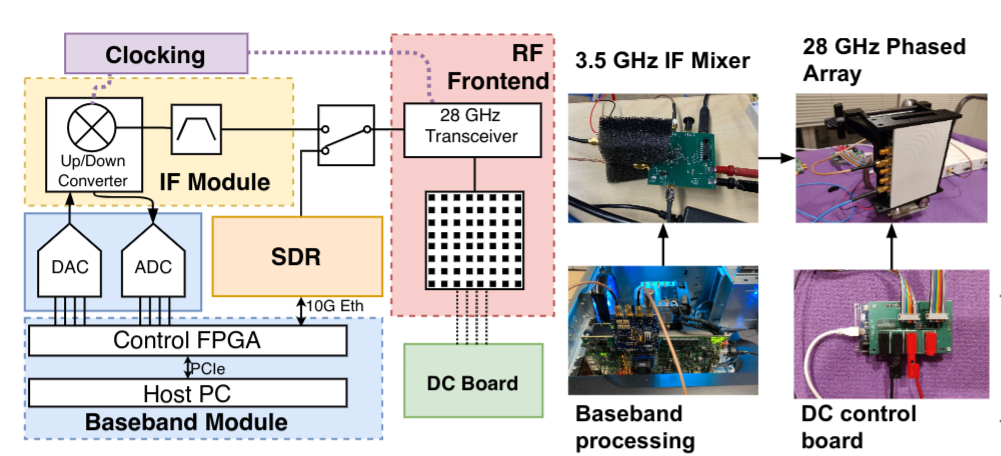
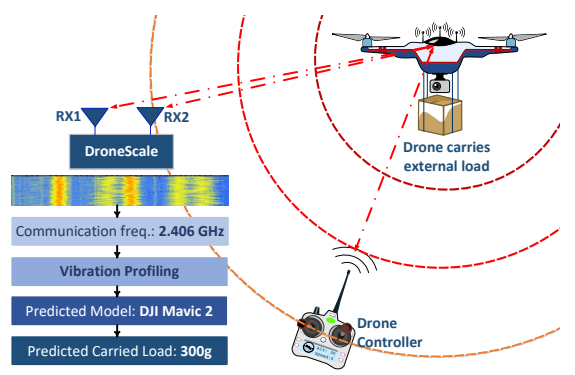
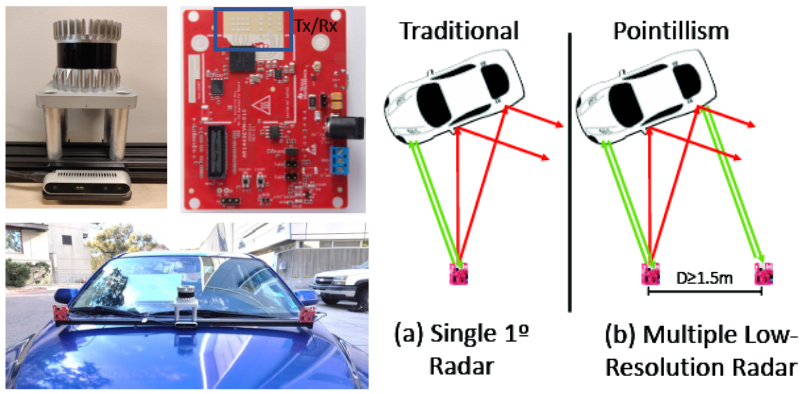
Open Source Code and Datasets
- [ Pointillism: Accurate 3D Bounding Box Estimation with Multi-Radars Code ]
- [ Pointillism: Accurate 3D Bounding Box Estimation with Multi-Radars Dataset ]
- [ mMobile: Building a mmWave testbed to evaluate and address mobility effects Dataset ]
- [ SIGNet: Semantic Instance Aided Unsupervised 3D Geometry Perception Code ]
Team
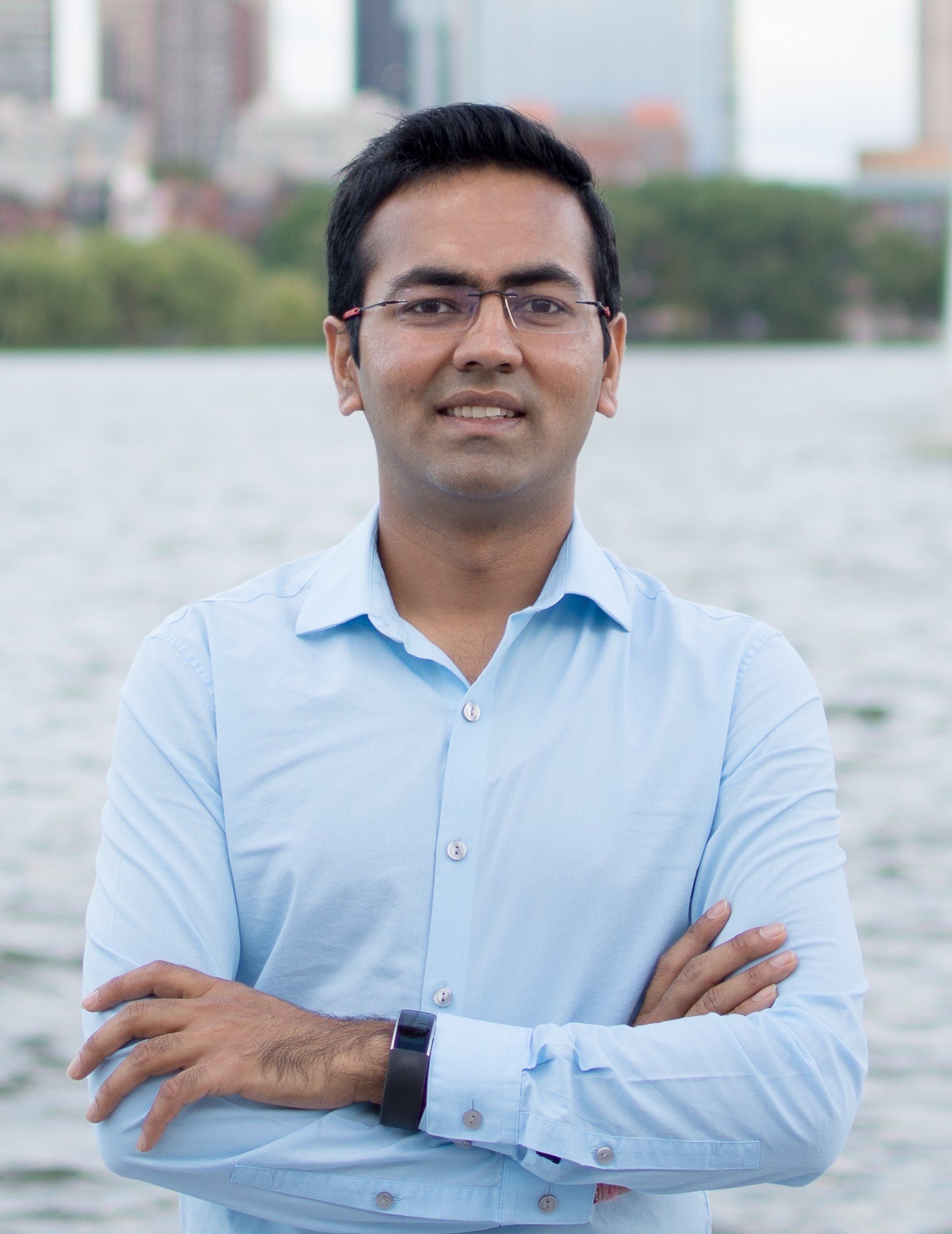
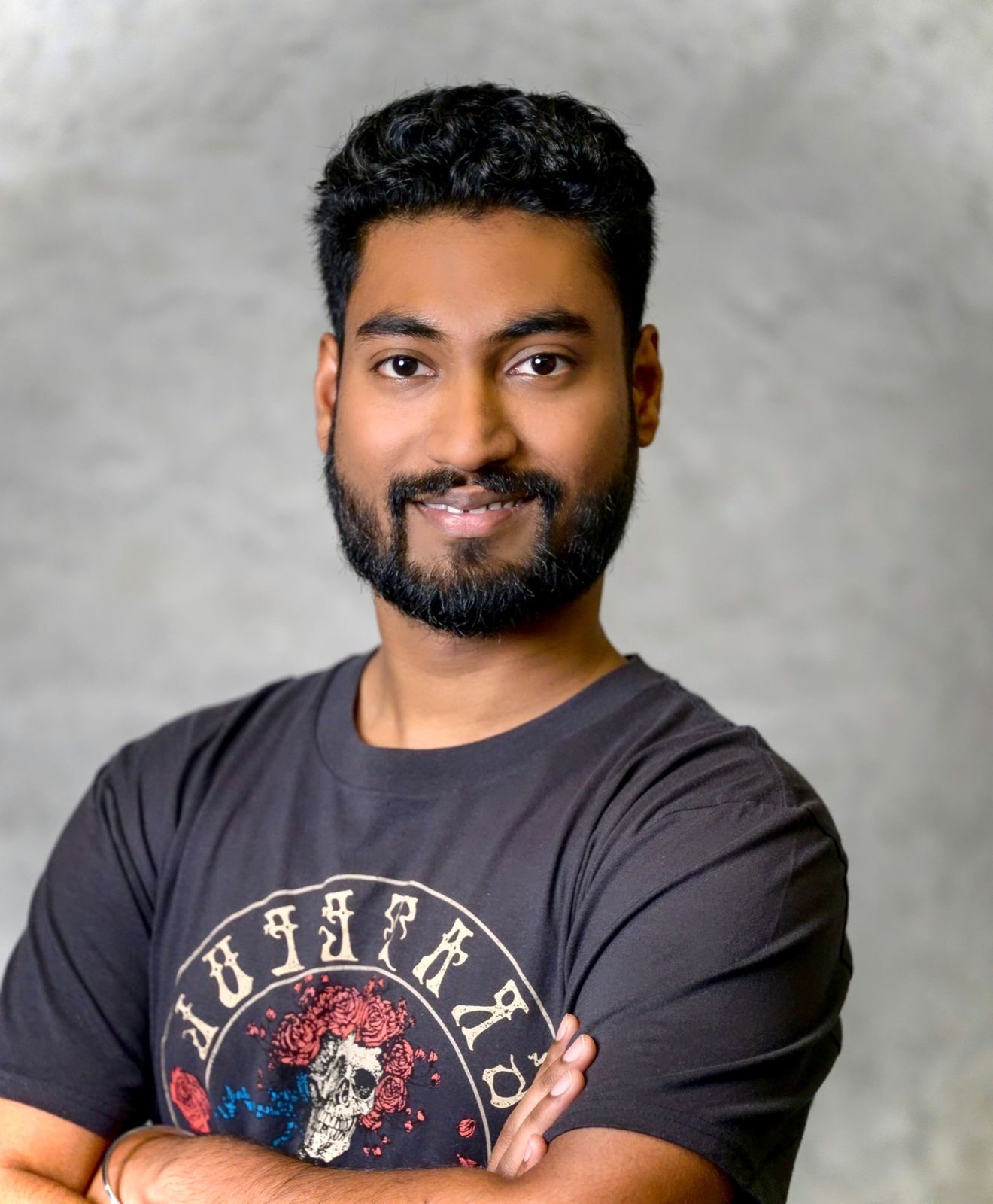